Study program / study programs: Advanced data analytics |
Course: Advanced data analysis in pharmaceutical research and development |
Teacher(s): Svetlana Ibrić, Ana Protić, Teodora Đikić, Biljana Otašević, Jelena Đuriš, Katarina Nikolić |
Course status: Elective |
ECTS points: 7 |
Prerequisites: none |
Course objective: Analyzing complex data in pharmaceutical research and development |
Learning outcomes: Students will acquire new knowledge and skills in the field of data analysis in pharmaceutical research and development. Knowledge of recent and advanced computational methods of rational drug design, which include ligand based methods (QSAR, pharmacophore analysis, virtual screening of ligands databases) or structure based methods (virtual docking, molecular dynamics). Students will master advanced theoretical methods and acquire skills for use of various programs for determining bioactive conformations of ligands, formation and validation of QSAR models, virtual screening, virtual docking, and molecular dynamics. Knowledge of creating in silico methods and optimal procedure for rational design, evaluation, and selection of novel drug candidates with improved pharmacological, physicochemical and pharmacokinetic properties. Students will understand how to apply the concept of quality by design (QbD) in pharmaceutical research and development; by defining the target drug quality profile (QTPP), critical quality attributes and process parameters (CQA, CPP) and by defining the design space. Students will be able to compare and select appropriate methods for advanced analysis of data generated during drug formulation and manufacturing development, including machine learning methods (neural networks, decision trees, self-organizing maps, etc.) and multivariate classification and regression methods. Students will be introduced with methods which are used in quality control of finished drug products with special concern about certificate of analysis. They will gather knowledge about different separation mechanisms in selected chromatographic systems in order to apply predictive mathematical models in description of retention behavior of analytes, as molecules with specific chemical structures. Based on that, they will be able to perform analysis of quantitative relationship between structure and retention behavior (QSRR study), to define optimal chromatographic conditions for quality control of drugs and to evaluate the significance of selected structural characteristics and physicochemical properties on retention of molecules in chromatographic system. |
Course structure and content: Introduction to data analysis in pharmaceutical research and development Ligands and targets databases Advanced computational methods of rational drug design In silico methods and optimal procedure for rational drug design The concept of quality design (QbD) in pharmaceutical research and development Critical quality attributes and process parameters (CQA, CPP). Design space. Computer methods for defining design space Advance data analysis in pharmaceutical research and development Advanced data analysis in continuous process verification in the pharmaceutical industry Application of different advanced computational methods for calculation of molecular descriptors, physicochemical, quantum chemical, topological and constitutional The use of experimental design in defining the experimental region aimed for the proper recognition of patterns in retention behavior Application of different statistical programs for building and testing of predictive QSRR models with the assistance of machine learning methods Basic familiarity with multivariate analysis (MLR) and machine learning algorithms (ANN, GBT, SVR) are welcome prior to entering the course. The basics of pharma science related to advanced approaches used within the drug research and development will be provided during the course. |
Literature/Readings: G. L. Partick ed. (2017) “An Introduction to Medicinal Chemistry“, 6th Edition, Oxford University Press UK. D. J. Abraham ed. (2010) Burger’s Medicinal Chemistry and Drug Discovery, 7th Edition, Volume 1: Methods in Drug Discovery, John Wiley&Sons, Inc. D. J. Abraham ed. (2010) Burger’s Medicinal Chemistry and Drug Discovery, 7th Edition, Volume 2: Discovering Lead Molecules, John Wiley&Sons, Inc. Victoria PhD F. Roche PhD, S. William PhD Zito PhD, et al. (2019) Foye’s Principles of Medicinal Chemistry, 8th ed. Williams DA, Lemke TL, editors. Baltimore: Lippincott Williams&Wilkins. Andrew R. Leach. (2001) Molecular Modelling: Principles and Applications 2nd Edition. Glaxo Wellcome Research and Development, Pearson Education. Guidelines, ICH Harmonised Tripartite. “Pharmaceutical development.” Q8 (R2) Current Step 4 (2009); “Quality risk management.” Q9 Current Step 5 (2006); “Pharmaceutical quality system.” Q10 Current Step 5 (2008) J. Djuris ed. (2013) Computer-Aided Applications in Pharmaceutical Technology, 1st Edition, Woodhead Publishing UK. R. Kaliszan. (2017) Quantitative structure property (retention) relationships in liquid chromatography, Chapter 23 in Liquid Chromatography: Fundamentals and instrumentation, 2nd edition. Elsevier Inc. L. Komsta, Y.Vander Heyden, J. Sherma. (2018) Chemometrics in Chromatography, 1st Edition, CRC Press, Taylor & Francis group, LLC. |
The number of class hours per week: Lectures: 5 Labs: 0 Workshops: 1 Research study: 2 Other classes: 0 |
Teaching methods: Individual and group work; lectures and labs |
Evaluation/Grading (maximum 100 points): Pre-exam requirements (Project, conceptual solution): 30 Project (implementation): 70 |
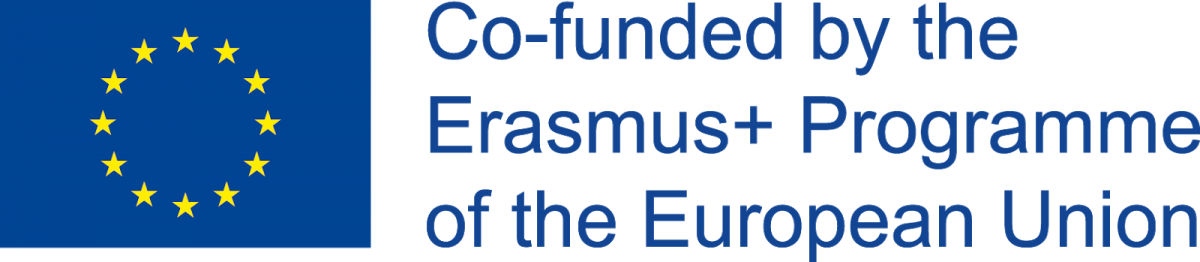