Study program / study programs: Advanced data analytics |
Course: Advanced data analysis in social sciences |
Teacher(s): Jelena Pešić, Jelisaveta Petrović, Dragan Stanojević, Irena Petrović, Oliver Tošković |
Course status: Elective |
ECTS points: 7 |
Prerequisites: Knowledge of basic statistical analysis techniques |
Course objective: Students will have the opportunity to familiarize with the advanced statistical methods and to perform analysis and interpretation of different datasets relevant for social science. The course concentrates on the practical application of advanced statistical methods and approaches in analyzing social world using cross-sectional, comparative, longitudinal, and panel datasets. |
Learning outcomes: Application of a range of advanced statistical methods on social science datasets: correspondence analysis, linear regression, ordinal linear regression, logistic regression, nonlinear models, multilevel, structural equations. Use of the statistical packages SPSS, STATA and R Interpretation of the statistical outputs. Writing reports based on advanced statistical analysis. |
Course structure and content: Introduction to practical advanced statistical modelling using social science datasets. Correspondence analysis. Linear regression. Ordinal regression. Logistic regression. Multinomial regression. Nonlinear models. Multilevel models – mix models. Structural equations modelling. This course assumes the students have basic knowledge of interferential statistics and basic skills in SPSS. |
Literature/Readings: Michael Mitchell, 2012, Interpreting and Visualizing Regression Models Using Stata, Stata Press. MacInnes, John, 2017, An Introduction to Secondary Data Analysis with IBM SPSS Statistics, Sage. Jasna Soldić-Aleksić, 2015, Primenjena analiza podataka. Rad u programima za statističku analizu i tabelarna izračunavanja, Ekonomski fakultet, Beograd Andy Field, 2013, Discovering Statistics Using IBM SPSS Statistics, 4th Edition, SAGE Publications Ltd. |
The number of class hours per week: Lectures: 5 Labs: 0 Workshops: 1 Research study: 2 Other classes: 0 |
Teaching methods: Individual and group work; lectures and labs |
Evaluation/Grading (maximum 100 points): Pre-exam requirements (Presentations of research project): 30 Final exam (Research paper – application of advanced statistical models on social science datasets): 70 |
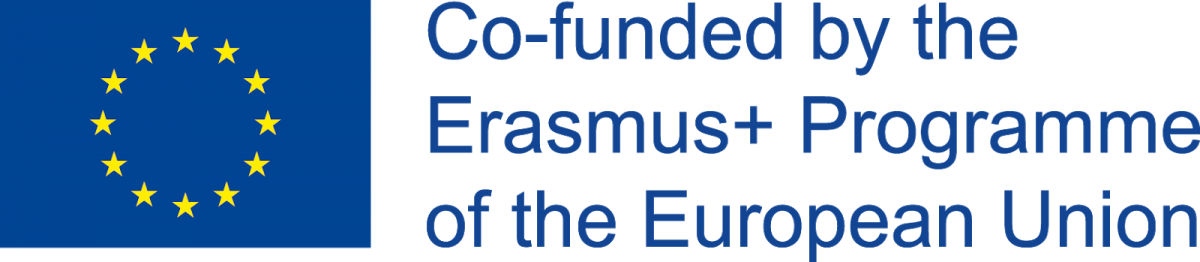