Study program / study programs: Advanced data analytics |
Course: Introduction to Statistical Inference |
Teacher(s): Bulajić Milica, Vukmirović Dragan, Radojičić Zoran, Jeremić Veljko, Dobrota Marina, Maričić Milica, Alorić Aleksandra, Mutavdžić Dragosav |
Course status: Elective |
ECTS points: 10 |
Prerequisites: none |
Course objective: Acquiring the ability to conduct statistical analysis, to interpret the obtained results, and to learn from the basic statistical methods and models how to study the observed phenomena as the subject of quantitative analysis. Getting acquainted with modern statistical software. |
Learning outcomes: After the course, student will acquire the basics needed for understanding the concepts of advanced data analytics in contemporary statistical software. |
Course structure and content: The concepts and techniques of statistical inference are first introduced through the review and presentation of theoretical foundations, followed by practical work using contemporary software. Introduction to statistics. Statistical set. Statistical feature. Sampling. Descriptive statistics. Collection, preparation and data visualisation Random variables and distribution models Statistics and parameters Estimation procedures. Point estimates. Confidence intervals. Hypothesis testing. Parametric tests. Nonparametric tests. Linear regression models. Simple linear regression. Multiple linear regression. Implementation of the covered methods and models in contemporary statistical software. |
Literature/Readings: Vuković, N. & Bulajić, М. (2014). Osnove statistike. Fakultet organizacionih nauka, Beograd. Mann, S. P. (2016). Uvod u statistiku. Ekonomski fakultet, Beograd. Field, A., Miles, J., & Field, Z. (2012). Discovering statistics using R. Sage publications. https://us.sagepub.com/en-us/nam/discovering-statistics-using-r/book236067 |
The number of class hours per week: Lectures: 4 Labs: 0 Workshops: 1 Research study: 2 Other classes: 0 |
Teaching methods: Individual and group work; lectures and labs |
Evaluation/Grading (maximum 100 points): Pre-exam requirements (Project): 60 Final exam (Oral exam): 40 |
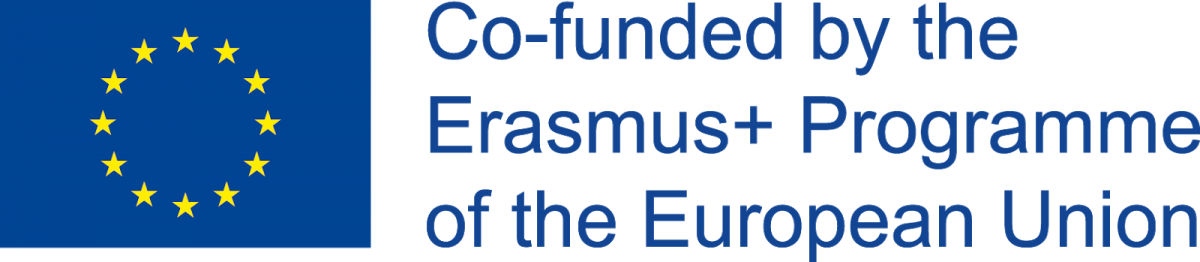